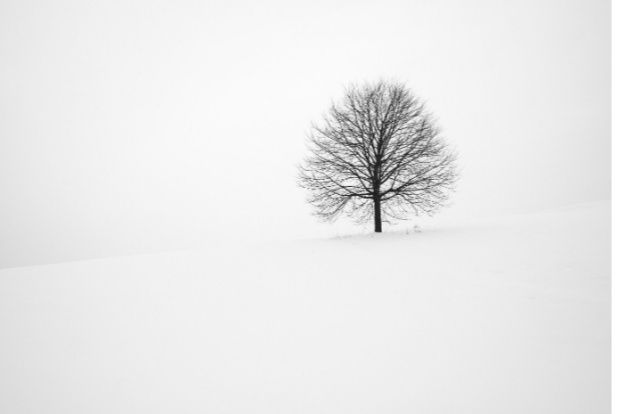
An AI winter speaks to a period where on the whole, positive sentiment towards opportunities in AI wanes, and most importantly, research and investments into AI effectively dissipates.
The first AI winter was in the mid ’70s, and was about a decade after the initial flurry of interest in artificial intelligence and machine learning. Majority of funding for AI was provided and spearheaded by the Defense Advanced Research Projects Agency (DARPA). It appeared that efforts made in the nascent field did not yield the expected results. Given that almost all funding was government backed, once the plug was pulled, it led to an era of little of no activity in the sector.
In the late ’80s, there was a renewed interest in AI with a greater focus on commercialized products and services. However there was significantly too much hype about the potential which backfired, and as such the interest waned and led to a second AI winter.

Since then, there has been a resurgence of AI from about 2010 which is sustained up until now.
➡️Another AI Winter?
There is general discussions around (see here and here) a third AI winter. Do I believe there is to be another AI winter? Yes and No at the same time…
➡️Why Not?
I believe this is unlikely for three main reasons:
Since the advent of big data, AI has become ubiquitous. The commercial applications of AI are all around us, and AI currently support both back end processes (e.g. robotics in supply chain), as well as customer/consumer facing applications e.g. chatbots, IoTs, etc.
Rapid strides in technology — accessibility to data, reduced cost of data and also immense processing power has enabled the improvements in AI over the years.
Currently, there is a diverse source of funding for AI — from both academia, the government and also commercial / private sector. One single driver is not able to create enough impact to drive interest down.
A mild chill, perhaps…
Whilst there is a much clearer distinctions as to what AI can do and what AI cannot do from a technical perspective, there is a further conversation to be had, and that is driving mainstream business value from AI. The business implementation of AI depends on a large number of non-technical factors such as
Overall human sentiment / perception about the technology and the dreaded mantra “AI is taking our jobs”
Required training and and retraining of staff to support AI implementation AI, and
Management’s (sometimes) negative view of AI especially as the outputs of AI algorithms support strategy and in certain cases trumps management’s preferred approach to business activities
These and more are the main dissenting factors impacting mainstream AI application and to the extent that these are not addressed, the large strides made in AI technology will not yield economic benefits and may ultimately suppress investments into the sector.
⚫Internet of Things (IoT): (usually) mainstream devices with sensors, processing ability, software, and other technologies that connect and exchange data with other devices and systems over the Internet or other communications networks. Source:
Examples include smart speakers, cctv cameras, connected doorbells, etc. IoTs are a major source of big data pulling information and data from the environment. Also a cause for concern from privacy and ethics perspective.