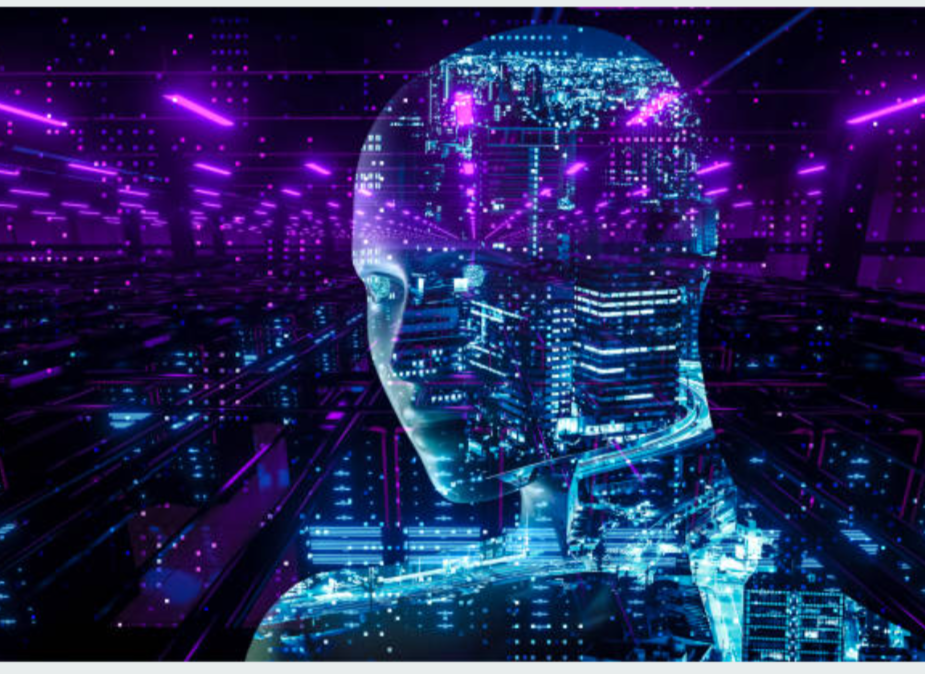
Machine Learning is often confused with artificial intelligence, but this is not true. An artificial intelligence technique that learns from data and provides results based on analysis is machine learning.
The expansion for data scientists will rise in the future as big data continue and grow. Machine Learning is a branch of artificial intelligence and computer science that focuses on the development of algorithms that replicate human learning processes in order to make machine learning better.
Data science is in large part built on machine learning and by applying statistical methods, algorithms are trained to make predictions, which can reveal important sights to data mining projects and ultimately these insights drive the decision-making process within applications and businesses resulting in greater growth.
WHAT IS THE MAIN COMPONENT OF MACHINE LEARNING?
Algorithms and Data are essentially the basis of machine learning but the data is what drives its success. In recent years machine learning has become increasingly popular thanks to deep learning and however without data, machine learning is not possible. Even if you have a good algorithm and if you do not have valid data you will not get excellent results.
CAN MACHINE LEARNING TAKE OVER OUR WORLD?
Science fiction movies create in most people's minds an image of Artificial intelligence as humanity is found to be a threat by using this source o technology. Data can teach machines, but they are not as intelligent as humans to become conscious of their actions
EXAMPLE-The car by itself cannot change its operations if the driver of the car is sick until and unless it's TESLA, but it's not like everyone can afford Tesla.
EVER WONDERED HOW DOES NETFLIX AND AMAZON RECOMMEND RECOMMEDATIONS?
We can answer this question using machine learning algorithms. By using machine learning, you can create a model using patterns found in your data. The aim is to explore the different possibilities of parameter-defined models. The calculations involved in an explanation will be more complex, but the models should be more simple. When you have a large amount of data, you can choose a complex method.