content factory, workers are not breaking a sweat operating heavy machinery, but rather using data engineering skills and domain knowledge of industry and business to give shape and context to datasets. You need both data engineering and domain experts to realize machine learning's full potential. Here is what they do:

Gather the data and map it out..
Sometimes called “data wrangling," data engineering is all about pulling together data and molding it into something digestible. After all, data can be structured, unstructured, or ambiguous. You have to first get the information out of multiple systems in order to then build models to map the data's behavior – that's the first step.
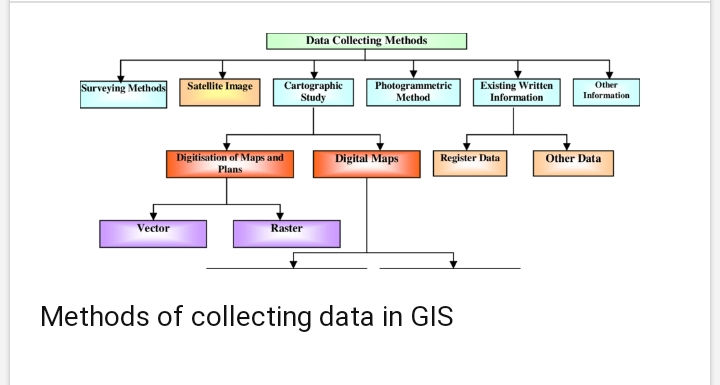
Find gaps in the information and fill them..!
You have to play with the data to find if it's complete and can serve the project goals, as there can be gaps in the information. For example, TV production companies need to figure out when to air ads based on time and audience. They typically have a sheet of data and tools that say what TV show to play an ad on and how long it should air. There might not be demographic and regional information. If they had such information, a company could use machine learning to determine which ads are the most effective by location and better target future placements.
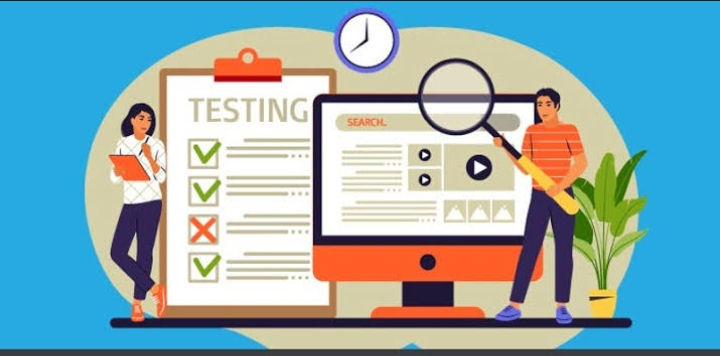
Show the machine the meaning..∆
Data enrichment is possibly the most complex and important piece of machine learning. It takes domain experts who understand how the business works to label the data with context and give it meaning.
Look for bias in data.*
Just like people, AI is prone to unconscious bias, stemming from imbalanced datasets or interactions over time. If left unchecked, biases can hurt your business and customers, ultimately defeating the purpose of turning to machine learning in the first place.
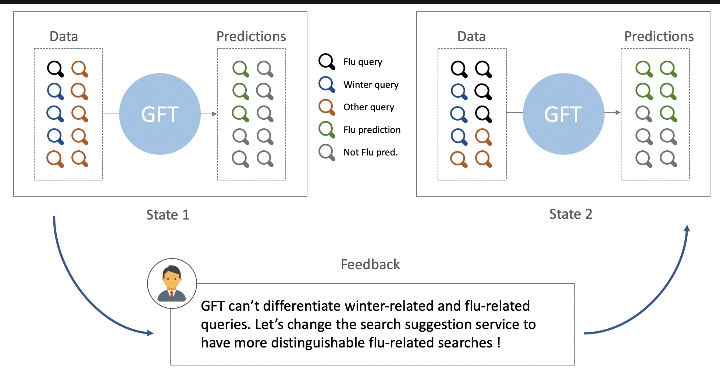
For instance, a company can use historical data to build a conversational AI model. But what if its past conversation records have been disproportionately with female customers? In that case, the model will have a gender bias to female customers. (Obviously, similar biases would come up if the model interacted with only male customers.) You need to review your data to get rid of existing biases, make sure the samples are comprehensive, and do ongoing reviews to prevent new biases.
All of these aspects of getting a machine learning project off the ground involve a lot of human work from people with data engineering and domain expertise that can enrich the data. So, while AI and machine learning can help you address your organization's toughest problems, people in the loop are vital to make it all work.